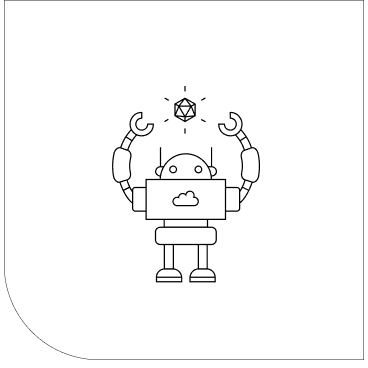
MICROSOFT Azure Machine Learning Workbench
Sr. Lead UX, Interaction & Visual Designer
2015 - 2016
Background
In the spring of 2016 I was the Lead Designer and manager of a small team of designers that were focused on a new exciting product - Azure Machine Learning Workbench, which was targeted for Data Scientists and Data Engineers. The work I'd done on Azure Data Catalog, Azure Data Factory, Azure Machine Learning, Cortana Intelligence Suite and Azure Enterprise Data Directory all had played a significant role in this new product.
PROJECT SCOPE
In mid 2015 our VP tasked the UX design team to develop a concept car around bringing a few key products in the Data Group together in a end-to-end big data analytics solution. Our design team developed a comprehensive deck that was presented and well received at a department all hands. I was a member of the original concept team and the work we did was the beginning of what would later become the Azure Machine Learning Workbench product.
The Workbench had gone through several iterations between 2015-2016 due to many factors - reorgs, product priorities, change in leadership, getting buy-in from leadership and a growing pressure from what was happening in the industry. After several reboots of the product concept and getting the right leadership consensus the product began to gain traction internally.
My involvement up to this point was primarily on developing UX and visual design concepts and helping with presentation decks for leadership to evangelize the product focus.
PROCESS
In the spring of 2016 the product began to have full support from our CVP and senior leadership. I was asked to lead the design efforts and manage a small team of designers, we would begin to bring the product to its first conception. I led numerous design sprints with our research, project management and lead engineer counterparts to begin to unravel some of the key concepts and scenarios we wanted to tackle first.
Whiteboard and paper concept sketches from one of the design sprint sessions.
During our daily sprints we began to learn a great deal about the product space, who our competitors were and who we thought our target personas were. We concluded that the product would be a great fit and candidate for a internal program called "Moneyball".
Leadership saw the potential value the Moneyball program would give the product team and we were accepted into the program. All disciplines - project management, engineering and design teams would be fully engaged on a daily full time basis for six weeks. Our primary focus would be to develop hypothesizes around particular aspects of the work process of a Data Scientist and prove them valid or not. We would talk to as many customers as possible and in the process we developed a insightful understanding of their work process.
During the six weeks we would hold two in-depth customer days where we would invite Data Scientists to join us for full day workshops in Redmond. We conducted a series of collaborative research sessions to understand their work habits, process and pain points.
During our customer days we ran numerous research exercises.
During the first half of the program we captured the Data Scientists work flow. During the second half of the program we used the above graphic to validate our assumptions of the Data Scientists process and to better understand their areas of pain.
Once the Moneyball program ended we took all the leanings, feedback and consolidated into a comprehensive road map that key stakeholders would form task groups around to tackle and develop "I cans" and key scenarios for the product.
My team would work with each of the task groups to develop UX vignettes to begin to unravel these key scenarios.
Interaction prototype developed in Proto.io to demonstrate early concepts.
In parallel to the scenario efforts my team was also responsible for developing a product UX framework and style guide. We worked closely with our product engineering team and project managers to understand key requirements and technical restrictions. All designs were developed in Sketch in combination with the Zeplin plugin to deliver accurate redlines to our engineers for implementation. Any particular interactions that we needed to demonstrate to our engineering team would be prototyped using either Invision or Proto.io.
Outcome
While I was still on the team, feedback from leadership was great and the team was scheduled to release Private Preview in Spring 2017. The project eventually went to market as Azure Machine learning operations (MLOps). I had left the team before then to join Microsoft Research (MSR) to head up the Computational Computer Vision (CV) design team and began to work on Azure Cognitive Services - Custom Vision.